Document Type
Article
Publication Title
Computers & Industrial Engineering
Abstract
In the automotive business environment, with intense competition among firms and with the added element of what is known as the “chips or semiconductor shortage,” adequate demand and supply chain planning is not forthright as semiconductors are essential to fabricating printed circuit boards, which in turn are necessary to control numerous computerized requirements of the vehicle. This paper presents a manufacturing postponement framework concerted with an ensemble forecasting method based on multi-layer Long Short-Term Memory networks combined with a bagging approach to predict highly fluctuating non-stationary printed circuit boards demand data in a business offering electronics manufacturing services to the automotive industry. The devised postponement framework effectively addresses the impending modifications required by the company to cope with the shortage of semiconductors. When the proposed forecasting method is embedded with the post-ponement framework, the procedure is called the Postponement Bagged Long Short-Term Memory (POST-BaLSTM) model. The approach aims to optimize semiconductor use, minimize wastage, and facilitate effective resource planning. The proposed method automatically fits the forecasts into the postponement structure by considering several Long Short-Term Memory models previously optimized using hyperparameters grid search. Bagged point forecasts and prediction intervals are derived using model estimates of quantile calculations. In our implementation, we demonstrate that a two-layered archetype using our method can lead to nearly a 43% improvement in measuring forecast errors compared to statistical and machine learning methods. Moreover, our approach bestows a 75% rating interval accuracy when measured with the denoted Spectrum Fidelity assessment.
DOI
https://doi.org/10.1016/j.cie.2023.109602
Publication Date
Fall 9-7-2023
Recommended Citation
Soto-Ferrari, Milton; Bhattacharyya, Kuntal; and Schikora, Paul, "POST-BaLSTM: A Bagged LSTM forecasting ensemble embedded with a postponement framework to target the semiconductor shortage in the automotive industry" (2023). Faculty Research Articles. 4.
https://scholars.indianastate.edu/scbfra/4
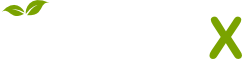
- Citations
- Citation Indexes: 4
- Usage
- Downloads: 15
- Abstract Views: 4
- Captures
- Readers: 32
- Mentions
- News Mentions: 1